Since the beginning of civilisation, some individuals have sought to profit from immoral and unethical practices. Nowadays, laws and regulations are set to curb criminal activity and prevent individuals from benefiting from wrongdoing.
In this article we present the constant struggle between malefactors and banks in the context of infrastructure and systems when it comes to hiding the source of fraudulent income: otherwise known as money laundering. It can be perceived as an arms race, in which both sides are caught in the Red Queen’s paradox*. We will then explore the possibility of using artificial intelligence (AI) to avert financial crime.
Money laundering and countering actions
What is money laundering?
It can be defined as:
- “transferring illegally obtained money or investments through an outside party to conceal the true source.” and
- “the processing of criminal proceeds to disguise their illegal origin”.
There are three steps to money laundering: placement, layering and integration. Placement can be considered the most difficult – the money must enter the financial system without triggering any alerts or raising suspicion. Legally operating firms and companies can serve this purpose and falsify their accounting to feed in the profits obtained through criminal activity. Alternatively, so-called money mules can transfer money on behalf of criminals for a commission.
Layering is a practice used often by criminals – during this process, the source of illegal money is, making it less possible to detect and track, so the money progressively starts to look more and more legitimate. The money is passed in portions, called “layers”, in transactions and via financial instruments. In the end, it seems to be a part of a legitimate finance flow.
Different techniques can be used to conceal the real source of illegitimate funds, for example:
- Forex transactions: changing the money’s currency
- Multiple inter-bank transfers
- Multiple wire transfers between different accounts in different countries
- Multiple structured deposits and withdrawals, which is also known as “surfing”
- Purchasing high-value items such as luxury goods (art, diamonds, jewellery, cars, real estate, etc.)
- Purchasing bonds and stocks
- Opening “shell”/fake companies
- Investing in businesses with minimal paperwork and with favourable regulations, such as currency exchanges, art galleries and car washes
- Exploiting loopholes like tax amnesty, trust companies, hiding ownership of a company or of specific goods
- Further using money “mules”
- Cryptocurrency to hide the source of funds
The last step in this process is integration. The money returns to the launder, is consolidated and seems unsuspicious, appearing as a legitimate transaction.
The cost of laundering
Financial crime is on the rise. According to the United Nations Office on Drugs and Crime, this accounts for around 3.6% of global GDP, or around $2.1 trillion, each year.
In 2018 it was estimated that the AML compliance costs for Europe only amounted to about €70 billion yearly. These costs increased by 21% between 2015 and 2017. One of its biggest components are employment and labour costs, both of which can be significantly reduced with the use of artificial intelligence (AI).
Artificial Intelligence
What is AI?
Techopedia provides the following definition: “AI is not a single technology. It is an umbrella term that includes any type of software or hardware component that supports machine learning, computer vision, natural language understanding (NLU) and natural language processing (NLP).” It can also be described as “the general ability of computers to emulate human thought and perform tasks in real-world environments, while machine learning refers to the technologies and algorithms that enable systems to identify patterns, make decisions, and improve themselves through experience and data.”
How AI can help in AML
AI can fill the gap in anti-money laundering (AML) needs. Banks and fintech companies constantly design and create new tools that can improve the techniques used for this purpose.
Money laundering can be detected in several ways using AI tools equipped with specialised algorithms with the goal of:
- analysing vast amounts of data;
- recognising patterns; and
- raising flags for unusual transactions or account activity.
AI can reduce false positives and negatives by effective transaction monitoring and alert investigation
Money launderers are using increasingly sophisticated methods to avoid detection and regulators are pressing for improved efficacy in AML programmes. The outcome of this pressure is also one of the common challenges of transaction monitoring and AML processes, which is the generation of numerous suspicious activity alarms. The estimate is that only 1-2% of these alerts are real threats while the rest are false positives. AI systems can support financial institutions in investigating these alerts and detecting and deactivating the false positives. This enables financial institutions to reserve more resources and time for handling the actual suspicious cases. The technology can also grade the risk level of threats, letting the investigators concentrate on the most suspicious and risky ones.
AI is also useful in detecting and reducing false negatives that usually occur due to ‘unknown unknowns’. This happens when the regulations for a new type of crime have not yet been formulated. AI solutions can perceive such anomalies and discover patterns that could not have been highlighted by existing rule-based logic. This is an essential functionality, as hackers use new technology to continuously create increasingly complex patterns.
In addition, AI could dramatically enhance the bureaucratic side of AML with its ability to automatically generate suspicious activity reports. Those documents can first undergo internal reporting and then be submitted to the relevant authorities. Moreover, the algorithms would pre-fill reports with relevant data and ensure standardised language and terminology. The time saved by this technology can be used by the compliance and AML departments to deal with suspicious activity.
AI increases efficiency in Know Your Customer (KYC) and Customer Due Diligence (CDD) procedures
AI algorithms are trained to detect suspicious behaviour in different ways. Also, due to the analysis of customers’ historical transactions, models can be put in place to help predict future behaviour, as well as to improve customer verification processes. The system is triggered even by subtle outliers in the pattern. The efficiency of AI in this task is higher than traditional AML systems.
Morever, AI has its advantages in the CDD and KYC processes, allowing both of these to be carried out faster and in more detail. Financial institutions can benefit from a greater range of customer data for AML purposes that can be used in risk assessments, suspicious activity reports, and in the case of investigations provided by AI.
Analysing unstructured and external data
Another advantage of AI is its ability to enhance the process of managing and editing unstructured data. The leverage is substantial, given that regulators require banks to analyse unstructured data as part of transaction monitoring, sanctions screening and more, to be AML compliant.
The amount of unstructured data required to ensure successful KYC and CDD for AML purposes is enormous. To understand the personal, professional, social and political backgrounds of a customer, it’s essential that financial institutions implement a risk-based approach. The unstructured data required to fully understand clients is collected from media, public archives, social networks and open-source data sources.
Although the traditional name searching methods may provide matches in external data, they will not be able to indicate the context or the possible relations and patterns. At that point,AI technologies with significantly higher processing power than the human brain greatly help with analysing the unstructured data and pointing out the connections and risks, which is a vital feature for AML processes.
One of the ways of getting the most out of unstructured data is the use of NLP. Its goal is to allow computers to process and analyse large amounts of natural language data, meaning that it would allow us to accurately extract meaningful information and quickly categorise large amounts of unstructured data.
Sufficient development of NLP would mean that AML and KYC procedures could be much more efficiently performed than with the use of traditional methods.
Some associated risks
AI, like everything else, is not perfect and prompts various risks, which could compromise results and, therefore, its efficiency:
- Input data may be out of date, incomplete and irrelevant. There is also the possibility of inconsistencies between the data used to train the algorithm and the actual input data during the operation. Additionally, a lack of available data may limit the scope of algorithms.
- Algorithm design can be threatened by coding errors, incorrect assumptions and biased logic.
- Output decisions can be misinterpreted and misused.
The underlying factors that affect the above risks are:
- Human biases: cognitive biases of model developers or users
- Technical flaws: lack of conceptual validity and consistency in training, testing and validation of the algorithm
- Usage flaws: errors in the implementation of the algorithm, its integration with operations
- Security flaws: the threat of gaining access to input data, output data or algorithm by unauthorised internal or external actors
Defining the end-to-end process – which includes planning, designing, building, testing and maintaining – will minimise risks. It is worth setting minimum requirements for data quality and access, as well as limiting downloads of sensitive data. A good technique is to mask personally identifiable information before it can be used in models. It is also important to monitor the environment in real time, maintain the ability to react quickly to a potential threat and agile disaster recovery.
While machine learning does not solve the issue of money laundering all by itself, it is a tool that greatly improves the efficiency and efficacy of AML processes. It is our belief that properly implemented machine learning solutions will soon become nearly indispensable, reducing the ever-rising costs of AML regulatory compliance. However, it must be understood that for a tool to fulfil its potential, it needs to be handled by an expert. Machine learning solutions are no exception.
“Now, here, you see, it takes all the running you can do, to keep in the same place. If you want to get somewhere else, you must run at least twice as fast as that!”
Money Laundering disruption remains a challenge
… but it can be solved more and more efficiently with AI.
Share this post
Written by
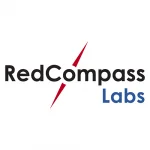
RedCompass Labs