When the steam engine first arrived, there was no standard way to describe its power. So Scottish inventor James Watt used a familiar metric: horses.
At the time, horses were widely used to pull carts, plow fields, and power machinery in mills. Watt wanted to show that his steam engine could do two things:
– replace horses, and
– do more work than people.
To make this clear, he calculated the power of an average horse based on how much work it could do over time. He estimated that a horse could turn a mill wheel of a certain size at a certain speed, doing about 550 foot-pounds of work per second. He called it horsepower.
The term stuck. Horsepower is still used to describe the power of cars, motorcycles, and farm equipment — even though horses are rarely used for transport anymore.
Today, we find ourselves at the dawn of another revolution, facing a similar challenge.
People power
Just as Watt tried to quantify power in terms people understood, today we need to understand how to quantify AI’s power when it fills in for people.
Take a project that, in 2025, would require 25 analysts. How do we describe it in the AI world? If two analysts and 23 AI agents complete the same work, do we call it a “25-people-power project”?
Do we price it based on the original 25?
Or do we reduce the price and charge more per head for AI-enabled analysts? They are, after all – the ones who orchestrate, review, and sign off the work.
What if we scrap this thinking and focus on fixed fees instead?
Today, a bank makes procurement decisions by comparing day rates, person-days, and project scopes. Whether it’s Prince2, PMP, or Agile, it still comes down to the same thing: How much work can a person do, and how many people will I need?
Big banks still require third-party analysts to be named individually in contracts. They calculate costs based on headcount and daily rates. Even with fixed-price contracts, they often reverse-engineer costs into a per-person model.
Right now, software companies charge for “users,” even if the “user” is just an automated file upload from a bank. There’s no human involved, but they still sell user licenses. This model could easily be applied to AI agents. The concept is not new. It’s just not widely understood.
So maybe AI takes the software approach. That would help it to align with current procurement models, Enterprise Resource Planning systems, and IT access controls. Projects could be priced based on the ‘users’ involved, whether human or agent.
But then again—does it matter if people aren’t involved at all? Agents do not have mouths to feed. They don’t need to sleep or vacation or worry about paying their mortgage. If project outcomes are all that matter, then surely procurement models and infosec rules need to adapt.
What happens to pricing?
Either way, AI could send the payments modernization model in a race to the bottom. If my AI agents are cheaper than yours, I’ll charge less. But each project is different. Banks have bespoke requirements tied to technology-specific methods aligned with payment rails rules. Agents’ output will need to be relevant to a specific project. It’s not a simple ‘copy/paste.’
The spotlight then shines on the quality of the agents and the expertise of those training and overseeing them. In a market-driven model, AI should lead to a reduction in costs for the client. But assuming a greater proportion of the work is done by agents, margins can still increase for the hybrid agent / human expert team.
Pricing then becomes value-based. Teams are formed of people and agents working together. Trust and quality take center stage.
After all – 99% accuracy is not good enough in banking. An unforeseen and untested ampersand </&> in the wrong place is all it takes to knock over a payments system. At least in the short- and medium-term, success will still require AI-enabled human experts in the loop.
Agents in the workplace
So, what about onboarding? For every person working in a bank, there is a background check, risk assessment, and conflict of interest checks. There’s data protection, systems access, training, insurance and liability coverage, and performance monitoring. How does this translate to AI agents?
Large language models (LLMs) can read and write. To leverage their extraordinary skills, agents need dedicated environments and interfaces to interact with internal teams. They need access to systems like Microsoft Teams for real-time communication, platforms like Confluence and Jira for managing documents and project workflows, and Outlook (or similar) for email communication.
Just as human analysts undergo background checks and risk assessments, a bank’s information security (infosec) team will need guarantees that external agents are secure, auditable, and compliant with data protection regulations.
These – in theory – should remove friction from onboarding. Instead of waiting weeks or months for an analyst’s checks to come through, agents can be onboarded immediately. However, only if clear agent infosec rules and protocols are adopted by clients and providers. This means that third-party AI tools will need validation processes to ensure they adhere to internal policies.
Conflict of interest checks will evolve into model bias evaluations and assessments of training data sources. Instead of individual system logins, banks may require AI models to operate within controlled sandboxes.
Training for human employees will also shift, focusing on how to interact with AI tools securely and ensuring liability is clearly defined when AI is informing, supporting or making decisions.
Performance monitoring won’t just measure output quality but will track drift in AI models, flagging inconsistencies or unexpected behavior. Banks will also look for ways to measure productivity gains from AI while maintaining regulatory compliance.
So, what will it be?
To truly unlock the potential of AI, banks must totally rethink how they price, procure, and onboard third-party services.
Either we continue using human equivalency as a benchmark, or we fully transition to a fixed-fee model.
We must move from per-person contracts to models that account for the power and efficiency of AI agents. That will mean reimagining how AI agents are integrated, from data protection to performance monitoring, ensuring that AI and humans can work seamlessly together.
Do we shift away from human-based metrics and start valuing the outcome—how much work is done, not who does it?
Whichever path we take will set the stage for how AI-powered work is valued, shaping everything from pricing models to project workflows in the coming years. But for that to happen, we – as the payments industry – need to start thinking about AI more maturely.
This is the third article in our “The Last Consultant” Special Report. If you missed the first two, you can catch up on how AI is reshaping the payments industry here (The Last Consultant and How AI is reshaping the labor force).
Share this post
Written by
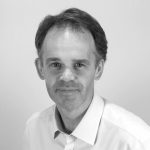
Tom Hewson
CEO, RedCompass Labs