It used to take a whole village to harvest oats in Shetland. Now one person can do it. Look at the Midwest or any breadbasket around the world. What used to take hundreds of laborers – just a few generations ago – now takes one person in a combine harvester. With autonomous machinery, guided by GPS, it takes none.
I’m sure the first combine harvesters broke down a lot, and I’m sure the first cars did, too. We know the first PCs were annoying. None of those things should make us think that the uptake of AI will be any easier.
You can question its ability. We’ve all seen answers that don’t make sense. But like combine harvesters, cars, and PCs, AI is moving in one direction: toward ubiquity. AI is improving every day. It will be better than humans at many things in a matter of months. Very soon, we are going to see technical documentation generated by AI that is of higher quality than we see today – across almost every project in the financial world.
In January 2025, UK Finance published a report that explored the software development life cycle (SDLC). They said two things: one, they’re already seeing 50-60% increases in speed and efficiency from generative AI within banking when it comes to developing software. Very generic. That could be upfitting your payments platform, integrating with new fintech, rolling out new products, or upgrading your core banking. Whatever that is, UK Finance said to the industry, “We are already seeing 50 to 60% acceleration from the use of generative AI.”
They also said, “You should prepare for the impact of that on your workforce.” That is a strong statement to slot into a case study of SDLC reviews. The message to consultants and analysts is clear: Prepare.
This is coming to an end. No one is quite sure what the future holds. The pace of change is happening, not in a five— to– 10-year cycle like before, but in one-to-two.
The river only flows one way
Last May, I stood in a payments workshop with a leading Canadian bank. I said, “In 36 months from now, your world is going to be utterly transformed.” The room was sceptical at best. Today, I am embarrassed. It’s happening much faster than that.
Six months later, we went back. The Head of Payments said, “I don’t know what analysts are going to do.”
What, in two years? Three years?
“No. Now. With AI, I don’t know what they’re going to do.”
The job most consultants do is low-hanging fruit for Large Language Models and Generative AI – reading rulebooks, creating epics, and writing user stories. The fact that a consultant could read 200 reports and connect the dots between them was once amazing. Now, it’s nothing.
Think about it. Paying a consultant to type is a waste of money. Their value is not in time spent tapping away at a keyboard – it’s in the knowledge they carry in their heads. If AI can free them from doing mundane tasks, consultants will have more time to work on high-value strategic work and better serve their clients. That’s a really good thing. But, just like early combine harvesters, cars, and PCs – most AI models are not ready yet.
Fine-Tuning
This is because general AI tools lack precision. And in payments, 99% right is not good enough. Payments experts ask complex, nuanced questions that need exact answers. Answers that broad AI models, like ChatGPT, cannot guarantee.
ortunately, “fine-tuning” can help. With fine-tuning, we take a broad AI model, like Llama or GTP4o, and tune it with payments-specific data sets. The model is given everything there is to know about payments– context, regulations, internal processes. And, with something called Cognitive Task Analysis, it can understand the subtle nuances of expert thinking. That makes it far more accurate for payments-related tasks than a broad model.
A fine-tuned AI model can understand things about payments that a general AI such as ChatGPT can’t. It knows how a payments expert would interpret a rulebook, a set of regulations, and a specific payments problem. It knows how they think. It can read between the lines to deliver accurate and contextually aware responses.
So, what does this mean for consultants and analysts?
Consultants and analysts can use these fine-tuned AI models as assistants for their payments projects. A single consultant can employ multiple AI agents, each with a specific task, to work on their behalf. One agent will coordinate a workflow, another will analyze the problem, and another will retrieve and prepare the documents. The agents review, test, and even score each other’s work to ensure accuracy. They operate around the clock, taking on repetitive, time-consuming tasks, which allows the so-called Last Consultants (or analysts) to focus on higher-level, strategic decisions. Once the agents are done, their work is sent to the consultant for review, edits, and approval, before the agent publishes it to wherever it needs to go.
What used to an army of junior consultants weeks is now complete in a matter of minutes. That’s the landscape we’re operating in. Not in a year or six months. Today.
Reaping rewards
Where does this leave consultants, analysts, and other project members? The optimist would say that AI helps them to work faster and smarter than before, enabling more innovation in the dynamic payments space. The pessimist would say most of the project team is no longer needed to fulfill today’s workload. Either way, big changes are afoot.
However, before banks and purveyors of banking and payments can use AI to save millions on payments modernization projects as a result of the productivity gains, they’ll need to fundamentally shift their approach to AI to take advantage of them.
We see two major shakeups currently in motion:
- A shift in pricing, procurement, and onboarding for AI agents
- The shift from general AI to Applied AI
We’ll explore each in greater detail in the next set of articles.
Share this post
Written by
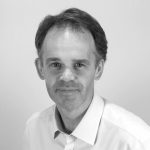
Tom Hewson
CEO, RedCompass Labs