There are more potential variations in a single ISO 20022 payment than there are atoms in the universe.
Hard to believe, isn’t it? But it’s true.
The dense network of metadata, rules, and interlocking systems that make up a single payment demands a level of precision—and a tolerance for complexity—that few other domains can match.
AI can help to make sense of it all, but not all models are useful.
Take, for example, general-purpose (or ‘horizontal’) AI, such as ChatGPT. ChatGPT has wowed the world by performing open-ended tasks, but it struggles in specialized fields like payments modernization. Yes, it recognizes terminology and can predict answers with some precision. But it currently lacks the structured logic and decision-making frameworks to make it useful for complex problems.
Even if future advances address this shortcoming, general AI solutions will continue to lack information hidden behind firewalls. They’ll also miss the ‘dark matter’ of the subject matter expert (SME) world: the 99% of knowledge and tradecraft that lives in an SME’s mind – not contained on the internet.
Vertical AI, on the other hand, taps into the minds of subject matter experts to provide highly accurate, dependable answers. But what is Vertical AI? How does it work? And how can we apply it to payments?
The Vertical AI advantage
Vertical AI are specialist AI systems designed for and applied to specific, complex industries. Vertical AI models don’t just generate text or summarise information. They capture explicit knowledge (rulebooks, standards, system intricacies, etc.) and implicit reasoning (cognitive processes, decision-making, understanding of nuance, interdependencies, and edge cases) to think like an expert.
So, how does it work? Vertical AI builds on top of existing large language models (LLMs), such as OpenAI’s GPT, DeepSeek etc, by adding a layer of domain-specific expertise. Think of it like fitting a high-precision lens onto a powerful camera—it sharpens the focus on payments, enabling the AI to reason with industry-specific knowledge and handle complex, nuanced scenarios.
One such model is AnalystAccelerator – a Vertical AI solution specifically designed for payments modernization projects. AnalystAccelerator doesn’t just generate text; it applies expert reasoning, aligned to the structured logic of a payments expert.
AnalystAccelerator has been created with:
- Fine-tuning (with real-world expert data) – The underlying AI model is trained on a mix of complex real-world payments examples. Payments experts provide scenarios and solutions so the model can internalize payments-specific rules, workflows, interdependencies and edge cases. This process takes thousands of hours, but the results are repeatable, scalable and worth it for the improvements in quality and accuracy.
- Continuous Pre-Training (using the largest global library of payments documentation) – We continue the foundation model’s broad training using our comprehensive annotated library of every rulebook and guideline covering every payment scheme in the world.
- A Proprietary Agentic Retrieval Framework: A specialized Retrieval-Augmented Generation (RAG) approach tailored specifically for the payments industry, addressing the challenge of accurately retrieving relevant documents from extensive resources. By integrating advanced techniques, the solution ensures that the retrieved data is precise, domain-aligned, and practically actionable.
- Cognitive Task Analysis (CTA) – A time-consuming method that takes thousands of hours of manual expert work to generate annotated datasets that can be used in a variety of post-training and fine-tuning approaches to help the AI understand how subject matter experts (SMEs) think and make decisions in complex scenarios.
By combining these components, AnalystAccelerator is trained to reason, think, and behave like a payments analyst.
To understand how it works, let’s explore these concepts in more detail.
1. Fine-tuning (with expert-curated, real-world data)
Pre-training provides foundational knowledge for a model. Fine-tuning takes it a step further by embedding domain expertise at a deeper level. We apply this to AnalystAccelerator using a hybrid approach:
- Expert-driven annotations and Q&A generation – Our payments experts feed the model thousands of anonymised, real-world Q&As from over 500 complex payment projects with banks. We then combine these with the experts’ annotations on payments rulebooks to create even more questions and answers for the model. This includes spotting edge cases (rare or unusual scenarios) within a document to make sure the examples align with real-world client use cases.
- Human expert validation – SMEs review all AI-generated outputs, making sure they are 100% accurate and meet industry best practices. The expert then provides quantitative feedback to the model, strengthening its fine tuning.
- Iterative feedback loop – The model is continuously refined based on the expert’s corrections and real-world usage.
This boosts accuracy, drastically reduces hallucinations, and delivers better contextual awareness compared to generic LLM fine-tuning approaches.
For example, AnalystAccelerator 2.5 benchmarks 13% higher in output accuracy, quality and completeness for payments-specific tasks when compared to Chat GPT-o1.
2. Domain adaptation through Continued Pre-Training (using the largest global library of payments documentation)
Continued pre-training is the process of further training an already pre-trained foundation AI model on additional domain-specific data to extend its capabilities or align it more closely with specific domains.
Continued pre-training is resource intensive and needs to be done each time the foundation model for the vertical AI solution is upgraded, or new approaches to payments modernization are discovered.
AnalystAccelerator undergoes continued pre-training using every rulebook, standard and regulatory update in the world ensuring:
- The foundation model is trained specifically for payment-related tasks and outputs.
- New regulatory updates are included in the model’s training.
- Evolving industry best practices from RedCompass Labs’ SME network are captured
This approach ensures the model can perform highly specialized tasks, far outpacing foundation models. As one measurement of domain specificity, AnalystAccelerator’s domain adapted model achieves perplexity scores ten times lower than the foundation model when tasked with payments-specific work.
3. Agentic Retrieval Framework for payments excellence
Having such a large pool of documents enables AnalystAccelerator to be comprehensive, but it also creates a challenge in ensuring the most relevant and accurate documents are referenced when performing complex tasks
Taking a proprietary and specialized approach to Retrieval-Augmented Generation (RAG), tailored specifically for the payments industry, significantly enhances the accuracy, completeness, and reliability of AI-driven outputs
AnalystAccelerator’s agentic retrieval framework integrates multiple retrieval mechanisms including, Hypothetical Document Embeddings (HyDE), Multi-query retrieval, Named Entity Recognition (NER), and lexical optimization – all of which have been fine-tuned for the payments domain
These sophisticated retrieval mechanisms ensure that the data the model uses to generate its output are contextually precise and domain-aligned. The Agentic Retrieval Framework consistently retrieves highly accurate, verifiable information, ensuring outputs are not only well-referenced but also practically actionable for payments teams.
By prioritizing domain expertise over general knowledge, the solution provides deeper, context-aware insights that align closely with real-world payments processes, standards, and regulatory requirements
As a result, the proprietary agentic retrieval framework provides payments professionals with trustworthy, comprehensive, and error-free outputs, minimizing risk in critical modernization initiatives.
4. Cognitive Task Analysis
One of the greatest challenges in AI for financial services is capturing not just what experts know, but how they think. Cognitive Task Analysis (CTA) solves this by dissecting how human subject matter experts think, reason and make decisions.
By combining the payments pre-trained model with ‘cognitive architectures’, the limitations of one (for example, payment-specific knowledge but no understanding of how to interpret rare or unusual scenarios) are complemented by the strengths of the other (the understanding that A could be interpreted as XYZ). CTA involves:
- Pairing skilled knowledge curators with subject matter experts to break down complex analytical tasks into a list of decisions.
- Identifying heuristics (mental shortcuts or ‘rules of thumb’) used by expert analysts to handle edge cases.
- Mapping the steps, actions and questions that lead experts to solutions for regulatory compliance, risk mitigation, system interdependencies and so on.
- Generating comprehensive datasets of annotations that can be used for fine-tuning, pre-training and evaluation.
By embedding these reasoning pathways into the solution, AnalystAccelerator avoids the pitfalls of purely statistical AI models that can only parrot surface-level information.
Instead, the AI has data about the “why” behind payment modernization projects. It doesn’t just recognize patterns in payments language but understands the reasoning behind decisions.
(Image: A simplified view of the CTA process)
Vertical AI and payments modernization
Generic AI models will always fall short in high-stakes, deeply regulated environments like payments. Vertical AI, on the other hand, is a true accelerator for financial institutions navigating the complexities of payments modernization.
If your bank is facing payments system upgrades or regulatory challenges, now is the time to leverage expert-driven AI. Unlock the full potential of your payments team with a breakthrough solution that doesn’t just deliver the generic —it reasons, advises, and drives real impact.
Curious about how RedCompass Labs and AnalystAccelerator can support your payments modernization journey?
Share this post
Written by
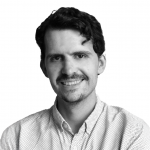
Alex Henry
Head of Product, RedCompass Labs
Resources